Since 1993
A Lucky Fool Doesn’t Know He’s Lucky, by Definition (Fooled by Randomness)
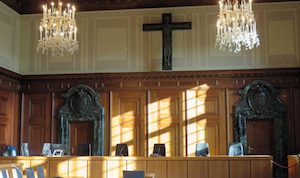
Every 23 seconds, an attorney is asked: “What are my chances of winning?”
Some attorneys play along with this game, especially if it’s a slow month, and throw out a number, like: “You have an 90% chance of winning”. Most people call several attorneys, and the attorney who is most confident (throwing out numbers, say, in the 90% range) may end up getting the case. Confidence is a good for business, right? But, are such numbers honest?
After 23 years of defending criminal cases (my web people love statements that brag about decades of practice, I will get a star beside my name for this), I am here to tell you that such numbers are pulled out of thin air. I know, it’s shocking to hear that an attorney could utter words that stretch the truth. Pull yourself together and read on, because other professions are just as guilty of spitting out misleading/unsubstantiated numbers as attorneys.
Financial advisers and scientists are at the top of the misleading numbers list. Yes, this is why you don’t believe every infomercial that claims “studies have shown xyz 99% effective”. But the problem here runs deeper than shady marketing practices, the problem involves what sort of conclusions scientists and financial analysts should be permitted to draw from their data. To understand problems with data interpretation, we’re going to take a journey through my Kindle highlights from an excellent book by Nassim Taleb entitled Fooled By Randomness. The book explains just how difficult it is to answer the very simple question “What are my chances”.
After many years of writing articles for this glorious website, you know that I rarely write about a book unless a couple of conditions are present. First, I have to be bored with writing legal stuff. Check. Second, the book has to be good. Fooled By Randomness is excellent. This “article” is not a book review, because book reviews take too much time and require a deeper command of the subject matter than I have. Well, technology has come to my rescue. Lucky for you, my Kindle eBook reader saves highlights of important concepts. I then cut & paste these highlights, for your reading pleasure. I hope that you learn as much as I did without having to read so much.
Doctors Are Bad With Numbers
What happens when thousands of patients are told by their doctor that they have a disease which they don’t have? The following is based on a true story (not like Fargo is, but really, this happened). It goes like this: A medical test is accurate 95% of the time, and gives false positive readings 5% of the time for a particular disease. “The disease strikes 1/1,000 of the population. People are tested at random, regardless of whether they are suspected of having the disease. A patient’s test is positive. What is the probability of the patient being stricken with the disease?” (Taleb, loc. 3352 of 4891 My Kindle doesn’t give me a real page number, sorry, you’ll have to suffer through my Kindle location numbers)
What are the chances the patient has this disease after testing positive? “Most doctors answered 95%, simply taking into account the fact that the test has a 95% accuracy rate.” Id.
The real answer is that the patient only has a 2% chance of having the disease. Here’s why:
Remember that 1 person out of 1,000 will have this disease. Now, remember that the lab test has a 5% false positive rate, meaning that, out of a 1,000 people, 50 people will test positive for this disease. So yes, the test is 95% accurate, sort of, but the test will show 50 out of 1,000 people as having a disease when we know the disease only afflicts 1 out of 1,000 people. If you open your calculator app and divide 1 person who really has the disease by the 50 people who will test positive for it, you end up with a 2% chance of having the disease after testing positive. Id. Yes, this means that thousands of people were told they had a disease which they did not have, all because doctors could not interpret data. You see kids, math really is important.
Again, what conclusions should we draw from certain statistics? While we’re picking on doctors, let’s say a surgical procedure has a 99% survival rate. What does this tell you? Put another way, what would you say to the doctor that claims “so far we have operated on ninety-nine patients with great success; you are our hundredth, hence you have a 100% probability of dying on the table.” Id. at 3436
The above “dying on the table” problem highlights the fact that there is confusion surrounding “unconditional and conditional life expectancy. At birth, your unconditional life expectancy may be seventy-three years. But as you advance in age and do not die, your life expectancy increases along with your life. Why? Because other people, by dying, have taken your spot in the statistics, for expectation means average. So if you are seventy-three and are in good health, you may still have, say, nine years in expectation. But the expectation would change, and at eight-two, you will have another five years, provided of course you are still alive. Even someone one hundred years old still has a positive conditional life expectation.” Id. [emphasis added]
How much can numbers tell us about causation? Clearly, data can show a correlation without any clear causation elements. Some philosophers have expressed doubt in our ability to ever deliver causation, and Fooled By Randomness explains how difficult it can be to prove causation, noting, if “hospital A delivered 52% boys and hospital B delivered the same year only 48%; would you try to give the explanation that you had a boy because it was delivered in hospital A?” Id. at 3467. You cannot look at Hospital A’s numbers versus Hospital B’s numbers and draw any sort of helpful conclusions.
Should We Trust Explanations of Financial Data?
Often, people strive to attach meaning to numbers that only represent noise. For example, say a cable news reporter states that the “Dow is up 1.03 on lower interest rates . . . the journalist claims to provide an explanation for something that amounts to perfect noise. A move of 1.03 with the Dow at 11,000 constitutes less than a 0.01% move. Such a move does not warrant an explanation. There is nothing there that an honest person can try to explain; there are no reasons to adduce. But like apprentice professors of comparative literature, journalists being paid to provide explanations will gladly and readily provide them.” Id. at 3455 [emphasis added]
It can be difficult to tease out which numbers are noise, and which numbers have meaning. Taleb explains that “[unless] something moves by more than its usual daily percentage change, the event is deemed to be noise. Percentage moves are the size of the headlines. In addition, the interpretation is not linear; a 2% move is not twice as significant an event as 1%; it is rather like four to ten times. A 7% move can be several billion times more relevant than a 1% move! The headline of the Dow moving by 1.3 points on my screen today has less than one billionth of the significance of the serious 7% drop of October 1997. People might ask me: Why do I want everybody to learn some statistics? The answer is that too many people read explanations. We cannot instinctively understand the nonlinear aspect of probability.” Id. at 3478.
If you’re still interested in these Fooled by Randomness topics, my favorite ideas from the book show up in my next ‘article’ (air quotes) “Proving All Swans Are White”.